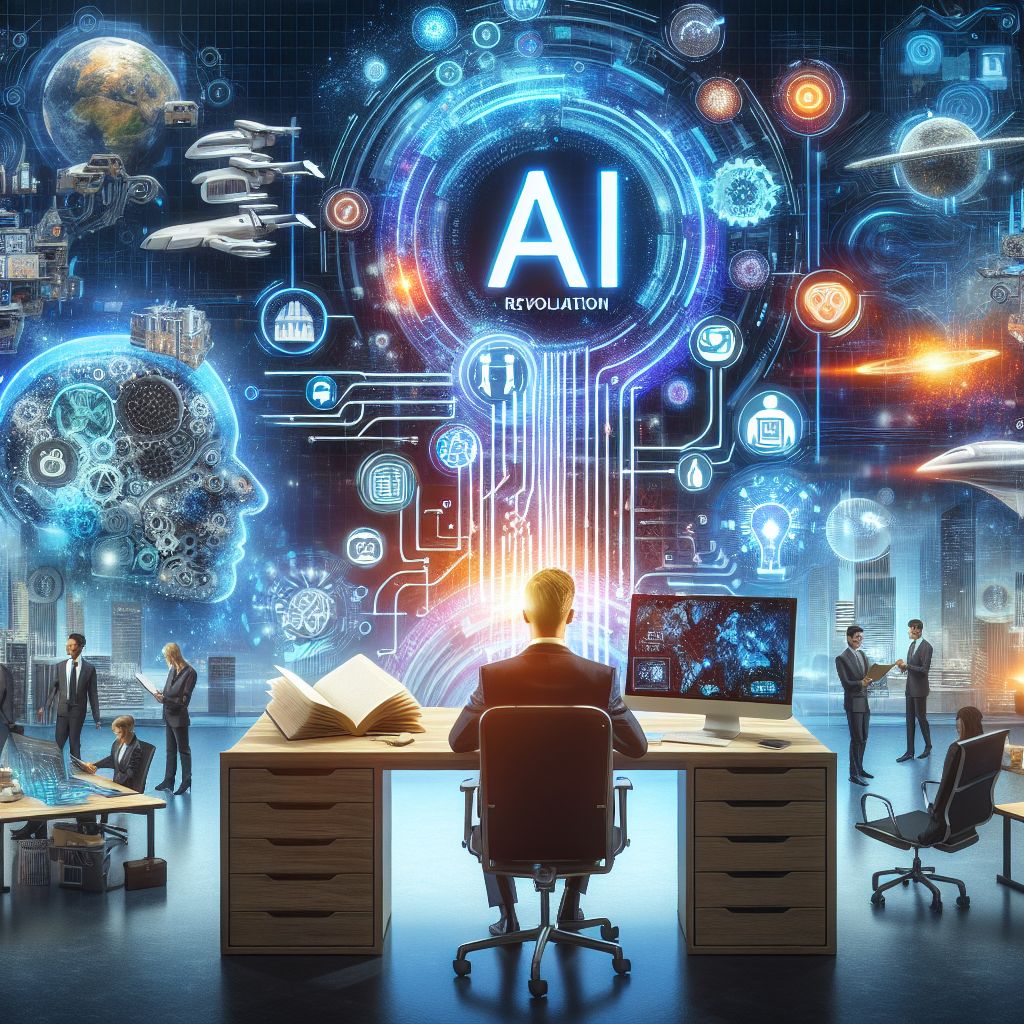
Decoding the AI Revolution: What Every Business Leader Should Know
Artificial intelligence (AI) is no longer a futuristic fantasy confined to science fiction. It’s here, it’s impacting every industry, and it’s rapidly reshaping the business landscape. For business leaders, understanding the fundamentals of AI is no longer optional; it’s a strategic imperative. While you don’t need to become a coding expert, grasping core AI concepts is crucial for making informed decisions about technology investments, process optimization, and future-proofing your organization.
This article provides a non-technical introduction to the essential AI concepts that every business leader should know, demystifying the jargon and empowering you to leverage AI’s potential effectively.
AI: More Than Just Robots
At its most basic, Artificial Intelligence refers to the ability of a computer system to perform tasks that typically require human intelligence. These tasks can range from simple calculations to complex problem-solving, learning, and even creative endeavors. However, the key distinction lies in the “intelligence” aspect. AI systems are designed to mimic human cognitive functions, such as:
- Learning: Acquiring knowledge and improving performance over time.
- Reasoning: Drawing conclusions and making decisions based on available data.
- Problem-Solving: Identifying and addressing challenges through logical analysis.
- Perception: Interpreting and understanding sensory input, such as images, audio, and text.
While robots are a common visual representation of AI, it’s important to understand that AI is the brain behind the operation. AI can power software, algorithms, and systems that operate independently of any physical form.
The Core Components: Machine Learning, Deep Learning, and Neural Networks
Within the broader field of AI, several key subfields are driving innovation. Understanding these distinctions is vital for appreciating AI’s capabilities and limitations:
- Machine Learning (ML): Imagine teaching a computer to learn without explicitly programming it. That’s the essence of machine learning. Instead of pre-defined rules, ML algorithms learn patterns and insights from data. The more data they’re exposed to, the better they become at predicting outcomes, making classifications, or generating recommendations.
- Examples: Spam filtering, fraud detection, product recommendation systems, predictive maintenance.
- Deep Learning (DL): A more sophisticated subset of machine learning, deep learning employs artificial neural networks with multiple layers (hence, “deep”). These layers process information in a hierarchical manner, allowing the system to learn complex representations and abstract features from data.
- Analogy: Think of how the human brain processes visual information. Our eyes capture raw pixels, which are then processed by different layers in our brain to identify edges, shapes, objects, and ultimately, understand the entire scene. Deep learning mimics this process.
- Examples: Image recognition, natural language processing, speech recognition, autonomous driving.
- Neural Networks: The foundational building block of deep learning, neural networks are inspired by the structure and function of the human brain. They consist of interconnected nodes (neurons) organized in layers. Each connection between neurons has a weight associated with it, representing the strength of the connection. By adjusting these weights during the learning process, the network can learn to map inputs to outputs with increasing accuracy.
- Simply put: Neural networks are like complex, interconnected spreadsheets that learn from data and adjust their calculations to achieve a desired outcome.
The Fuel for AI: Big Data
AI, particularly machine learning and deep learning, thrives on data. The more data available, the better the system can learn and improve its performance. This is where the concept of “big data” comes into play.
Big data refers to extremely large and complex datasets that are difficult to process and analyze using traditional methods. These datasets are characterized by the “Three Vs”:
- Volume: The sheer amount of data.
- Velocity: The speed at which data is generated and processed.
- Variety: The different types of data (structured, unstructured, semi-structured).
Big data provides the raw material for AI algorithms to identify patterns, make predictions, and uncover insights that would be impossible to detect manually.
Practical Applications of AI in Business
AI is no longer limited to theoretical applications; it’s being deployed across a wide range of industries and business functions. Here are a few examples:
- Marketing: Personalized customer experiences, targeted advertising, predictive analytics for campaign optimization.
- Sales: Lead scoring, automated sales processes, chatbot-based customer service.
- Operations: Predictive maintenance, supply chain optimization, quality control.
- Finance: Fraud detection, risk management, algorithmic trading.
- Human Resources: Talent acquisition, employee training, performance management.
Navigating the Ethical Considerations
As AI becomes more pervasive, it’s crucial to consider the ethical implications. Key concerns include:
- Bias: AI systems can inherit and amplify biases present in the data they are trained on, leading to unfair or discriminatory outcomes.
- Transparency: Understanding how AI systems make decisions (the “black box” problem) is crucial for accountability and trust.
- Privacy: Protecting sensitive data used to train AI models is paramount.
- Job Displacement: The potential impact of AI on the workforce needs to be carefully managed.
Business leaders must address these ethical considerations proactively by establishing clear guidelines for AI development and deployment.
Key Takeaways for Business Leaders
- Embrace continuous learning: AI is a rapidly evolving field. Stay updated on the latest trends and technologies.
- Focus on business problems: Don’t implement AI for the sake of it. Identify specific business challenges where AI can deliver tangible value.
- Build a data-driven culture: Invest in data infrastructure and cultivate a culture of data literacy throughout your organization.
- Collaborate with experts: Partner with AI specialists and consultants to leverage their expertise.
- Prioritize ethical considerations: Develop a framework for responsible AI development and deployment.
In conclusion, understanding AI basics is no longer a luxury for business leaders; it’s a necessity. By grasping the fundamental concepts of machine learning, neural networks, and big data, you can make smarter decisions about technology investments, drive innovation, and future-proof your organization in the age of AI.
To truly unlock the power of AI in your business, a foundational understanding of its language is paramount. Dive deeper into the world of AI with The AI Business Dictionary: 200 Must-Know Words, Phrases, and Definitions. This eBook will equip you with the vocabulary you need to confidently navigate the AI landscape and make informed strategic decisions. Purchase your copy today at https://shop.mymobilelyfe.com/product/the-ai-business-dictionary-200-must-know-words-phrases-and-definitions/ and take the first step towards AI fluency.
Recent Comments