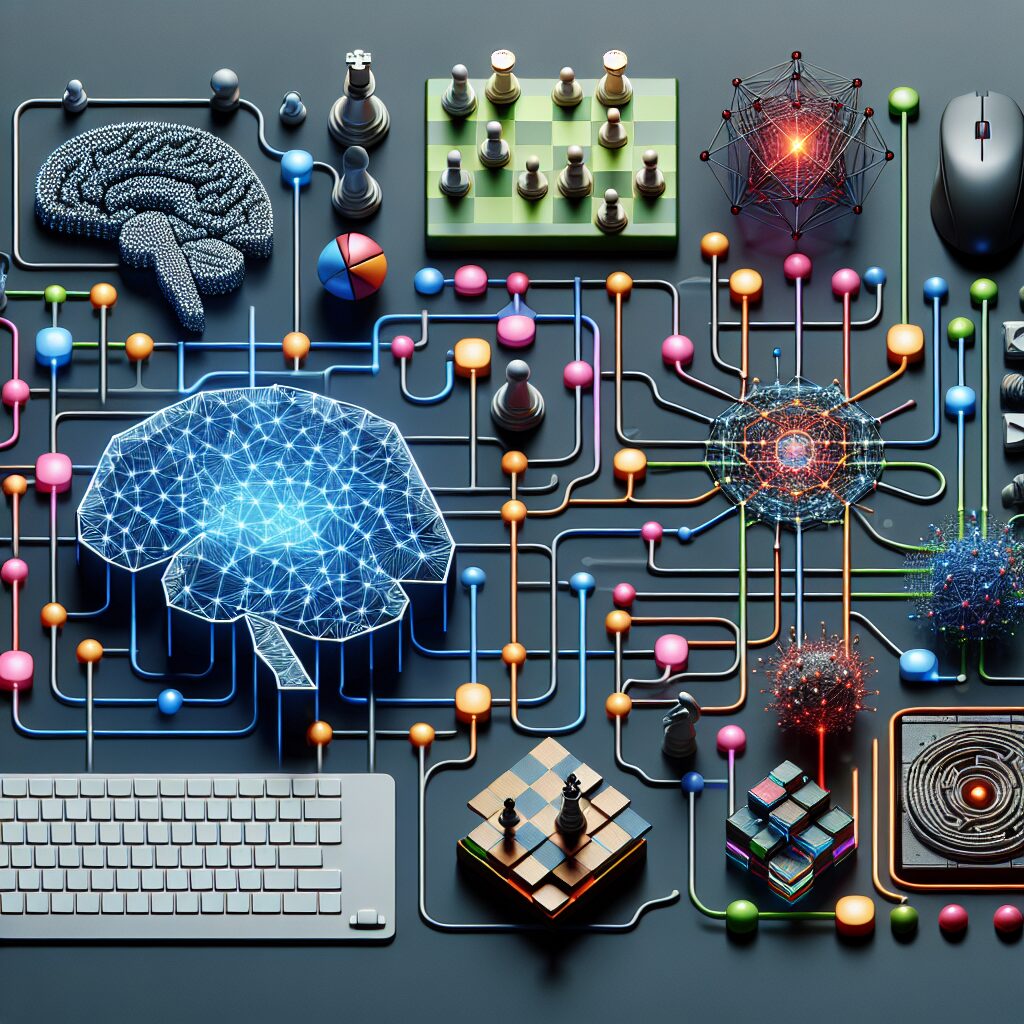
Building an AI Strategy That Actually Works: A Step-by-Step Guide
For COOs, CTOs, and innovation leaders, the allure of Artificial Intelligence (AI) is undeniable. The potential to streamline operations, enhance customer experiences, and unlock new revenue streams is compelling. However, many organizations find themselves lost in the hype, investing in AI initiatives that ultimately fail to deliver tangible results. The problem isn’t necessarily the technology itself, but rather the lack of a well-defined and actionable AI strategy.
This article provides a step-by-step guide to building an AI strategy that focuses on real business outcomes, moving beyond fleeting tech trends and delivering a sustainable competitive advantage. Forget chasing the shiniest object; it’s time to focus on the strategic implementation of AI that directly addresses your company’s key challenges and opportunities.
Step 1: Understand Your Business Objectives – Beyond the Buzzwords
The cornerstone of any successful AI strategy is a deep understanding of your existing business objectives. What are your core challenges? Where are the bottlenecks in your processes? What are your growth aspirations? Don’t start with “Let’s implement AI”; start with “What are our biggest pain points, and how can technology, possibly AI, help us solve them?”
Instead of focusing on AI for AI’s sake, consider the potential impact on key performance indicators (KPIs). For example:
- Increased Revenue: Can AI personalize product recommendations to drive sales, optimize pricing strategies to maximize profits, or identify new market segments for expansion?
- Reduced Costs: Can AI automate repetitive tasks to free up human resources, optimize supply chain management to minimize waste, or improve predictive maintenance to reduce equipment downtime?
- Improved Customer Experience: Can AI personalize customer service interactions, provide faster and more accurate responses to inquiries, or predict customer churn to proactively address concerns?
- Enhanced Operational Efficiency: Can AI optimize resource allocation, streamline workflows, or automate data analysis to improve overall productivity?
By connecting AI initiatives directly to these overarching business goals, you create a framework for measuring success and ensuring alignment across the organization.
Step 2: Identify AI-Ready Opportunities – Not Every Problem Needs an AI Solution
Once you understand your business objectives, the next step is to identify specific opportunities where AI can be applied effectively. Not every problem requires an AI solution. Sometimes, a simple process improvement or a more traditional technology solution may be more appropriate.
When assessing potential AI applications, consider the following factors:
- Data Availability: AI algorithms require large amounts of high-quality data to train and operate effectively. Do you have sufficient data available, and is it clean, accurate, and accessible?
- Problem Complexity: Is the problem complex enough to warrant an AI solution? Simple tasks that can be easily automated with rules-based systems may not require the sophistication of AI.
- Expected ROI: What is the potential return on investment (ROI) for each AI application? Prioritize projects that offer the greatest potential for generating value and align with your overall business strategy.
- Feasibility: Do you have the necessary resources, expertise, and infrastructure to implement and maintain the AI solution? Consider the costs of data acquisition, model development, deployment, and ongoing maintenance.
A useful framework for identifying AI-ready opportunities is the “AI Opportunity Matrix,” which plots potential AI applications based on their potential impact and feasibility. High-impact, high-feasibility opportunities should be prioritized, while low-impact, low-feasibility opportunities should be avoided.
Step 3: Define Clear Success Metrics – How Will You Know It’s Working?
Once you’ve identified potential AI applications, it’s crucial to define clear and measurable success metrics. These metrics will serve as a benchmark for evaluating the performance of your AI initiatives and ensuring that they are delivering the desired results.
Instead of vague goals like “improve customer satisfaction,” define specific and measurable metrics such as “increase Net Promoter Score (NPS) by 10 points within six months.” Similarly, instead of “reduce costs,” aim for “reduce operational expenses by 15% through automation.”
When defining success metrics, consider the following factors:
- Alignment with Business Objectives: Ensure that the metrics are directly aligned with your overarching business objectives and KPIs.
- Measurability: Choose metrics that can be easily tracked and measured.
- Timeframe: Establish a realistic timeframe for achieving the desired results.
- Baseline: Establish a baseline measurement before implementing the AI solution to provide a point of comparison.
Regularly monitor and track your progress against these metrics. If your AI initiatives are not delivering the expected results, be prepared to adjust your approach or re-evaluate your assumptions.
Step 4: Build or Buy? – Choosing the Right Implementation Approach
Once you have a clear understanding of your business objectives, identified AI-ready opportunities, and defined success metrics, you need to decide whether to build your AI solutions in-house or purchase them from a vendor.
- Building In-House: Building your own AI solutions allows you to customize them to your specific needs and retain control over your data and algorithms. However, it requires significant investment in infrastructure, expertise, and talent.
- Buying from a Vendor: Purchasing AI solutions from a vendor can be a more cost-effective and time-efficient option, especially for organizations that lack in-house AI expertise. However, it can also limit your flexibility and customization options.
The decision of whether to build or buy depends on a variety of factors, including your budget, timeline, in-house capabilities, and desired level of customization. Consider the following:
- Total Cost of Ownership: Evaluate the total cost of ownership for both options, including the costs of infrastructure, software, personnel, and ongoing maintenance.
- Time to Market: How quickly do you need to implement the AI solution? Building in-house may take longer than purchasing from a vendor.
- Level of Customization: How much customization do you require? Building in-house allows for greater customization, while vendor solutions may offer limited options.
- Data Security and Privacy: How important is data security and privacy? Ensure that your chosen solution meets your organization’s security and compliance requirements.
Step 5: Iterate and Optimize – AI is Not a One-Time Project
Implementing AI is not a one-time project; it’s an ongoing process of iteration and optimization. AI models need to be continuously trained and refined with new data to maintain their accuracy and effectiveness.
- Continuous Monitoring: Continuously monitor the performance of your AI models and track your progress against your success metrics.
- Feedback Loops: Establish feedback loops to gather insights from users and stakeholders and incorporate them into your model development process.
- Regular Updates: Regularly update your AI models with new data to ensure that they remain accurate and relevant.
- Experimentation: Experiment with different AI algorithms and techniques to find the best solutions for your specific needs.
By embracing a culture of continuous improvement, you can ensure that your AI initiatives continue to deliver value over time.
Step 6: Develop a Data Governance Strategy:
AI thrives on data, making a robust data governance strategy essential. This includes defining data ownership, ensuring data quality, and establishing policies for data privacy and security. Without a well-defined data governance strategy, your AI initiatives could be hampered by inaccurate, incomplete, or insecure data.
Step 7: Focus on Ethical Considerations:
As AI becomes more prevalent, it’s crucial to consider the ethical implications of its use. This includes addressing issues such as bias in algorithms, transparency in decision-making, and the potential impact on employment. Developing a strong ethical framework for AI development and deployment will help ensure that your AI initiatives are used responsibly and for the benefit of society.
By following these steps, COOs, CTOs, and innovation leaders can create an AI strategy that delivers real business value, rather than just chasing the latest trends. Remember, AI is a tool, not a magic bullet. It requires careful planning, execution, and ongoing optimization to achieve its full potential.
The complexities of building a successful AI strategy often require a unique leadership perspective. Uncover the secret ingredient many companies are missing. Learn more about the crucial role of leadership, even in unexpected places, in our eBook. Download The Invisible Chief AI Officer: Why Many Businesses Need a Leader They May Not See at https://shop.mymobilelyfe.com/product/the-invisible-chief-ai-officer-why-many-businesses-need-a-leader-they-may-not-see/
Recent Comments