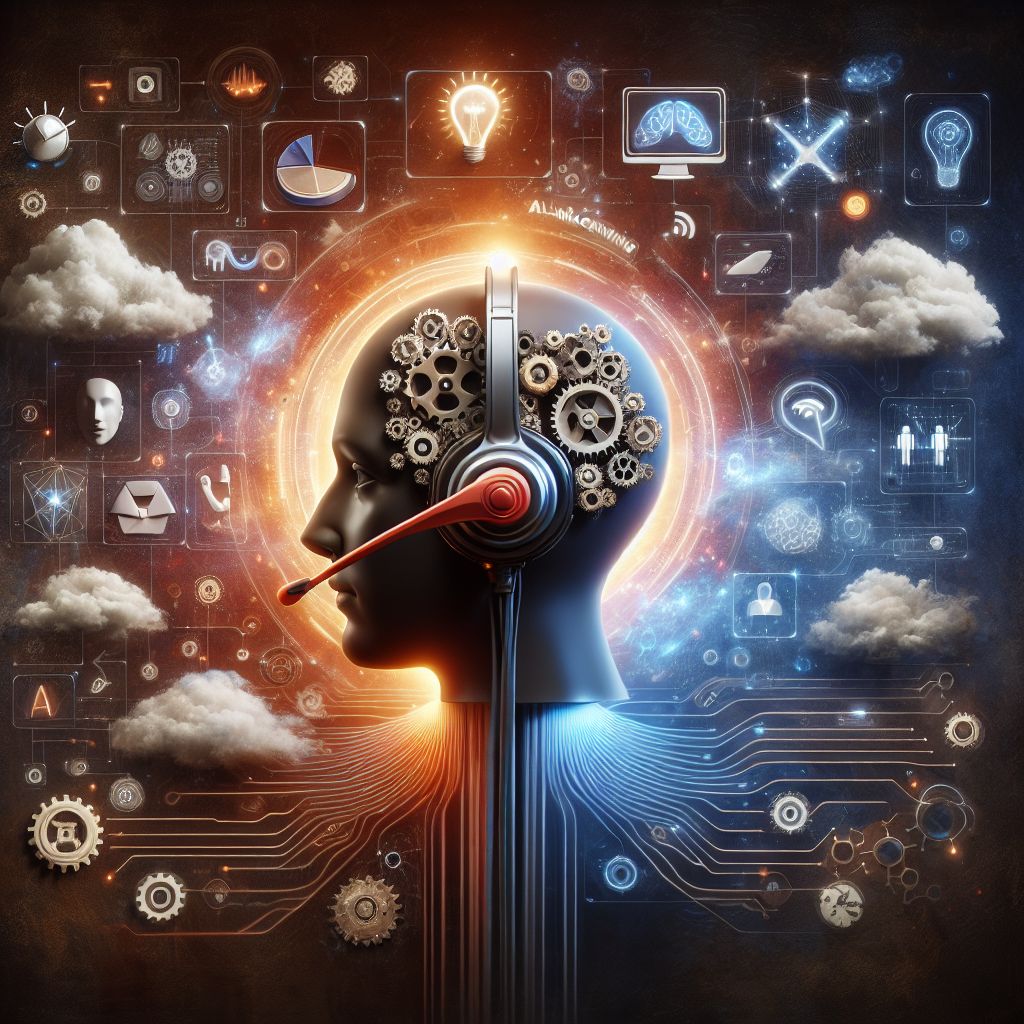
The Hidden Bias in AI: What Business Leaders Need to Know
Artificial intelligence (AI) is no longer a futuristic fantasy; it’s a present-day reality, rapidly transforming industries and reshaping the way businesses operate. From streamlining workflows to enhancing customer experiences, AI promises unprecedented efficiency and innovation. However, beneath the shiny surface of technological marvel lies a potentially treacherous problem: bias. AI systems, though seemingly objective, can unintentionally perpetuate and even amplify existing societal biases, leading to significant ethical and business risks. For business leaders, AI strategists, and compliance officers, understanding and mitigating these biases is not just a matter of ethical responsibility, but also a crucial step towards ensuring sustainable growth and long-term success.
The promise of AI lies in its ability to analyze vast datasets and identify patterns that would be impossible for humans to detect. However, the very foundation of an AI system – the data it is trained on – is often a source of bias. This is because the data we collect and use to train AI reflects the existing biases within our society. If historical data is skewed, the AI system will inevitably learn and perpetuate those skews.
Consider, for example, a hiring algorithm trained on a dataset of past employee performance. If that dataset predominantly features male employees in leadership positions, the algorithm may learn to favor male candidates, effectively perpetuating gender inequality. Similarly, a loan application system trained on historical data reflecting discriminatory lending practices could unfairly deny loans to individuals from marginalized communities.
These biases are not always conscious or malicious. In many cases, they are embedded within the data, often stemming from unintentional errors, historical prejudices, or simply a lack of diverse representation. This is precisely what makes them so insidious – they can creep into AI systems unnoticed, leading to unfair and discriminatory outcomes without anyone realizing the system is flawed.
Sources of AI Bias: Unveiling the Culprits
To effectively combat AI bias, it’s crucial to understand its root causes. Here are some of the most common sources:
- Data Bias: This is perhaps the most prevalent source of AI bias. As mentioned earlier, if the training data is not representative of the population it is meant to serve, the AI system will learn biased patterns. This can manifest in various ways:
- Historical Bias: Data reflects past inequalities and prejudices.
- Representation Bias: Certain groups are underrepresented or overrepresented in the data.
- Measurement Bias: The way data is collected or measured systematically favors certain groups.
- Algorithm Bias: Even with unbiased data, the algorithm itself can introduce bias. This can occur through:
- Feature Selection: The choice of which features to include in the model can inadvertently favor certain groups.
- Model Design: The mathematical models used in AI systems can amplify existing biases in the data.
- Optimization Criteria: The objective function used to train the AI system can prioritize certain outcomes that disproportionately benefit certain groups.
- Human Bias: Human decisions throughout the AI development lifecycle, from data collection and labeling to algorithm design and evaluation, can inject bias into the system. This can be due to:
- Confirmation Bias: Humans tend to seek out information that confirms their existing beliefs, leading them to inadvertently bias the data or the algorithm.
- Availability Heuristic: Humans tend to rely on readily available information, which may not be representative of the entire population.
- Unconscious Bias: Subconscious stereotypes and prejudices can influence decision-making, even when individuals are unaware of them.
Ethical and Business Risks: The Price of Ignoring AI Bias
The consequences of ignoring AI bias are far-reaching, impacting both ethical considerations and business outcomes.
- Ethical Risks: The most obvious risk is the perpetuation of discrimination and inequality. Biased AI systems can deny individuals access to essential services, such as loans, employment, or healthcare, simply because of their race, gender, or other protected characteristics. This not only harms individuals but also undermines the principles of fairness and justice.
- Legal Risks: Biased AI systems can violate anti-discrimination laws and regulations, leading to costly lawsuits and reputational damage. Companies that fail to address AI bias are increasingly likely to face legal challenges from regulatory bodies and individuals who have been harmed by biased AI systems.
- Reputational Risks: Negative publicity surrounding biased AI systems can severely damage a company’s reputation and erode customer trust. In today’s highly connected world, news of biased AI systems can spread rapidly through social media, leading to public outcry and boycotts.
- Financial Risks: Biased AI systems can lead to poor business decisions, resulting in financial losses. For example, a biased marketing campaign that targets the wrong audience can waste resources and damage brand perception. A biased risk assessment system can lead to poor investment decisions.
- Operational Risks: Biased AI systems can create operational inefficiencies and hinder innovation. If AI systems are not accurately reflecting the needs of all customers, they may not be effective in solving real-world problems. This can lead to wasted resources and missed opportunities.
Mitigating and Preventing AI Bias: A Proactive Approach
Addressing AI bias requires a proactive and multifaceted approach that spans the entire AI development lifecycle. Here are some key strategies:
- Data Auditing and Cleansing: Regularly audit training data for potential biases and cleanse it to ensure it is representative and accurate. This may involve collecting more diverse data, correcting errors, and removing irrelevant features.
- Algorithm Awareness: Be aware of the potential biases inherent in different algorithms and choose algorithms that are less susceptible to bias. Consider using fairness-aware algorithms that are specifically designed to mitigate bias.
- Fairness Metrics: Implement fairness metrics to measure the performance of AI systems across different demographic groups. This will help you identify and address biases that may not be apparent through traditional performance metrics.
- Bias Detection Tools: Utilize bias detection tools to automatically identify potential biases in data and algorithms. These tools can help you uncover hidden biases that you may not be aware of.
- Transparency and Explainability: Design AI systems that are transparent and explainable, allowing users to understand how decisions are being made. This will help you identify and address biases that may be hidden within the system.
- Human Oversight: Maintain human oversight of AI systems to ensure they are not perpetuating bias. This may involve setting up review boards to evaluate the performance of AI systems and making adjustments as needed.
- Diverse Teams: Build diverse teams of data scientists, engineers, and ethicists to develop and deploy AI systems. This will help you ensure that different perspectives are considered and that potential biases are identified early on.
- Ethical Guidelines and Training: Establish clear ethical guidelines for AI development and deployment and provide training to employees on how to identify and mitigate AI bias. This will help create a culture of ethical AI development within your organization.
By taking a proactive and comprehensive approach to addressing AI bias, business leaders can mitigate the ethical and business risks associated with this pervasive problem. Investing in bias mitigation strategies is not just a matter of social responsibility; it is also a strategic imperative for ensuring the long-term success and sustainability of your organization.
The Future of AI is Fair:
The future of AI hinges on our ability to build fair and equitable systems. By acknowledging and addressing the hidden biases within AI, we can unlock its full potential to improve lives and drive innovation. This requires a concerted effort from business leaders, AI strategists, and compliance officers to prioritize ethical considerations and implement robust bias mitigation strategies. The journey towards fair AI is a continuous one, demanding ongoing vigilance and adaptation.
Ready to take the next step towards responsible AI adoption? Learn more about how MyMobileLyfe’s AI services can help you recognize, mitigate, and prevent AI bias in your workflows. Visit us at https://www.mymobilelyfe.com/artificial-intelligence-ai-services/ to discover how we can help you build a more ethical and sustainable AI strategy.
Recent Comments